Grasp
Task-oriented Grasping: learning to use new tools and objects.
![]() |
Funded by the Belgian National Fund for Scientific Research (FRS-FNRS). |
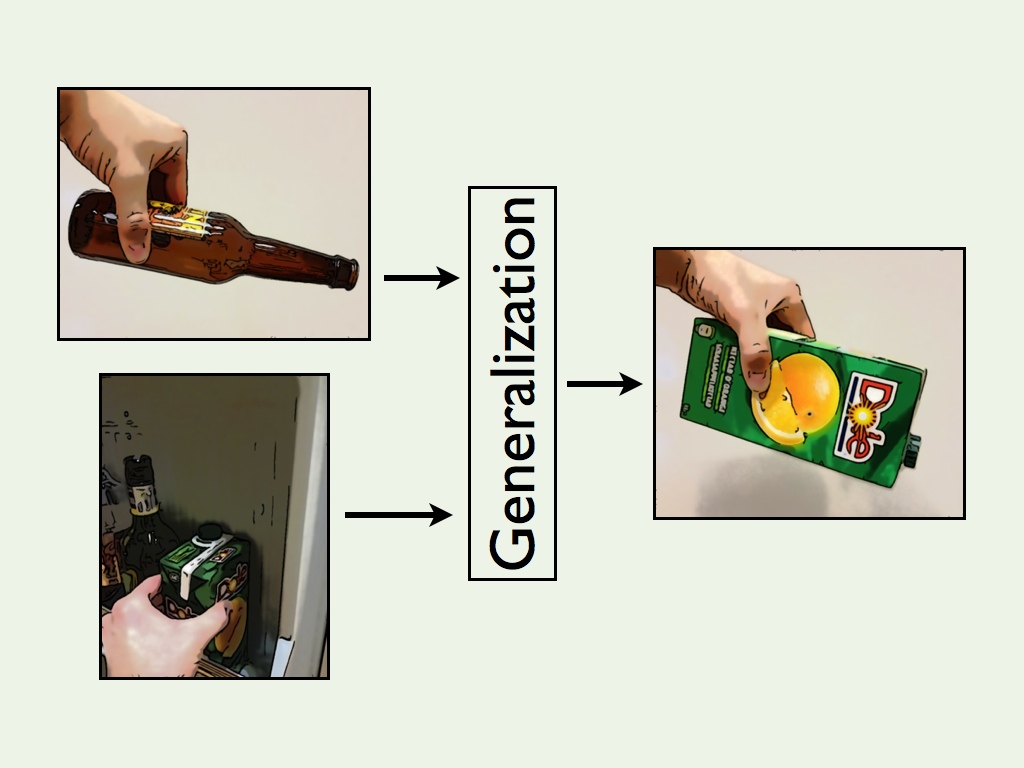
Task-oriented Grasping: learning to use new tools and objects.
![]() |
Funded by the Belgian National Fund for Scientific Research (FRS-FNRS). |
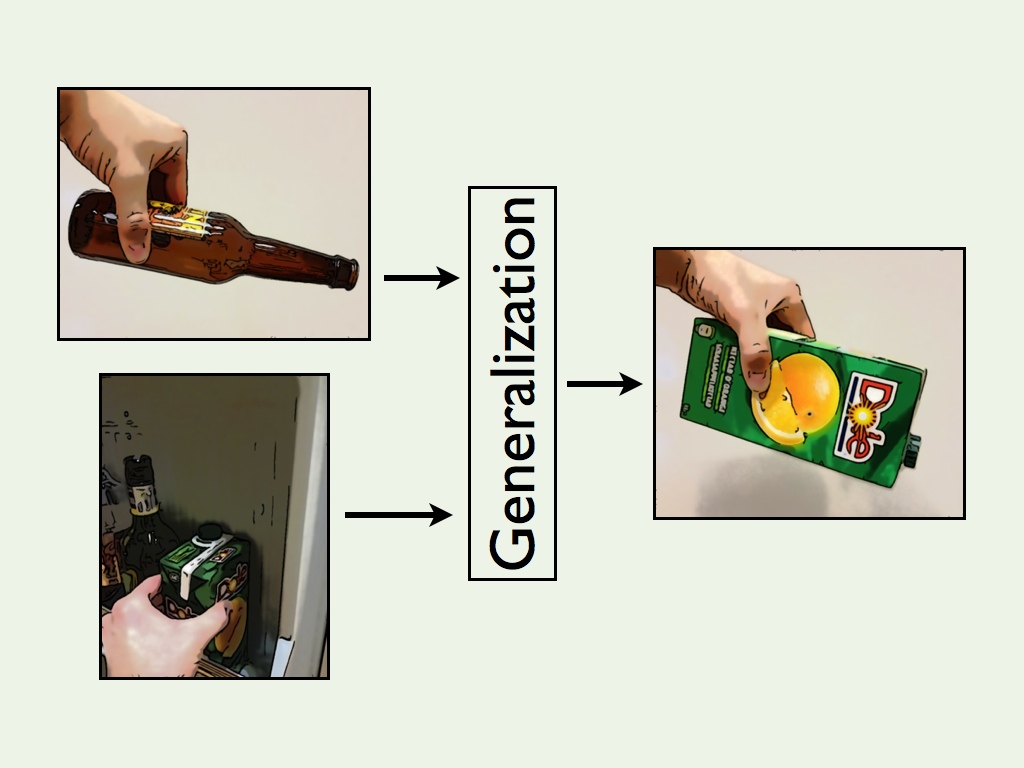
In this project, we address (1) the problem of grasping unknown objects, then (2) grasping new objects while respecting constraints imposed by a task.
Grasping New Objects
We present a real-world robotic agent that is capable of transferring grasping strategies across objects that share similar parts. The agent transfers grasps across objects by identifying, from examples provided by a teacher, parts by which objects are often grasped in a similar fashion. It then uses these parts to identify grasping points onto novel objects. While prior work in this area focused primarily on shape analysis (parts identified, e.g., through visual clustering, or salient structure analysis), the key aspect of this work is the emergence of parts from both object shape and grasp examples. As a result, parts intrinsically encode the intention of executing a grasp.
We devise a similarity measure that reflects whether the shapes of two parts resemble each other, and whether their associated grasps are applied near one another. We discuss a nonlinear clustering procedure that allows groups of similar part-grasp associations to emerge from the space induced by the similarity measure. We present an experiment in which our agent extracts five prototypical parts from thirty-two grasp examples, and we demonstrate the applicability of the prototypical
Task-oriented Grasping
We address the problem of generalizing manipulative actions across different tasks and objects. Our robotic agent acquires task-oriented skills from a teacher, and it abstracts skill parameters away from the specificity of the objects and tools used by the teacher. This process enables the transfer of skills to novel objects. Our method relies on the modularization of a task’s representation. Through modularization, we associate each action parameter to a narrow visual modality, therefore facilitating transfers across different objects or tasks.
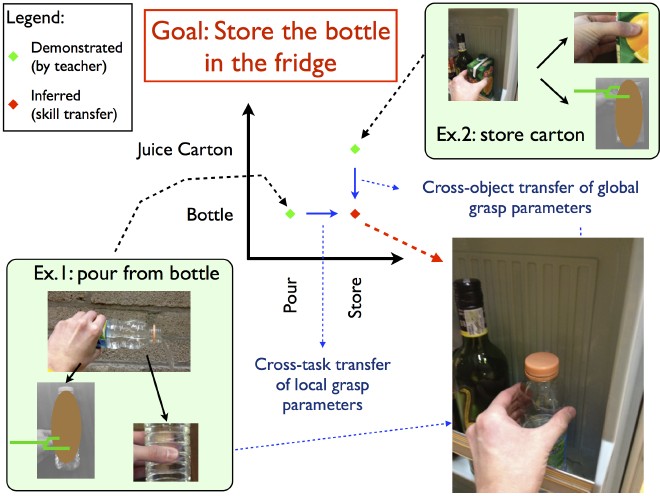