Plexegen
Grasping novel objects with tactile-based grasp stability estimates.
![]() |
Funded by the Swedish Research Council (VR). |
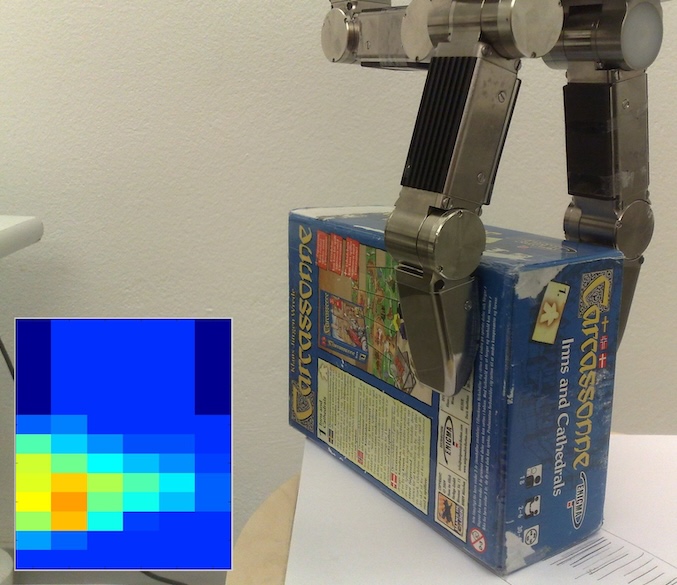
Grasping novel objects with tactile-based grasp stability estimates.
![]() |
Funded by the Swedish Research Council (VR). |
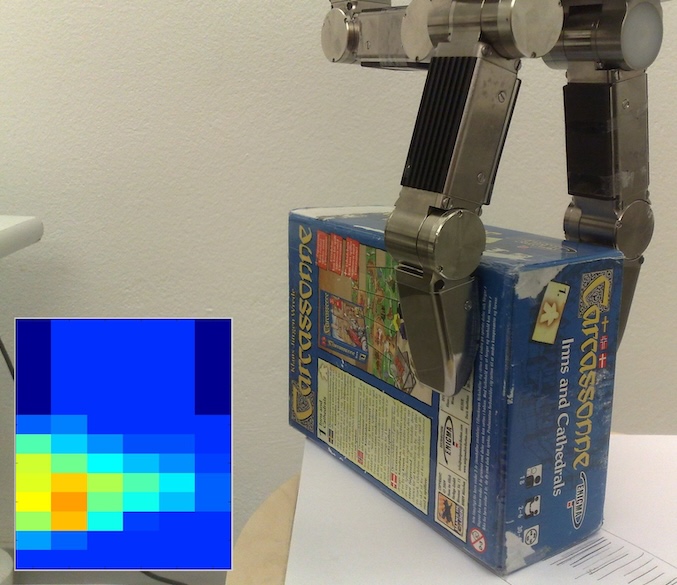
To grasp an object, an agent typically first devises a grasping plan from visual data, then it executes this plan, and finally it assesses the success of its action. Planning relies on (1) the extraction of object information from vision, and on (2) the recovery of memories related the current visual context, such as previous attempts to grasp a similar object. Because of the uncertainty inherent to these two processes, designing grasp plans that are guaranteed to work in an open-loop system is difficult. Grasp execution greatly benefits from a closed-loop controller which considers sensory feedback before and while issuing motor commands.
In this project, we study means of monitoring the execution of a grasp plan using vision and touch. By pointing a camera to the robot’s workspace, we can track the 6D pose of visible objects in realtime. Touch data are captured by sensors placed on the robot’s fingers. These two modalities are complementary, since during grasps objects are partly occluded by the hand, and visual object cues become uncertain. Monitoring the execution of a grasp allows the agent to abort grasps that are unlikely to succeed, thus preventing potential damage to the objects or the robot.
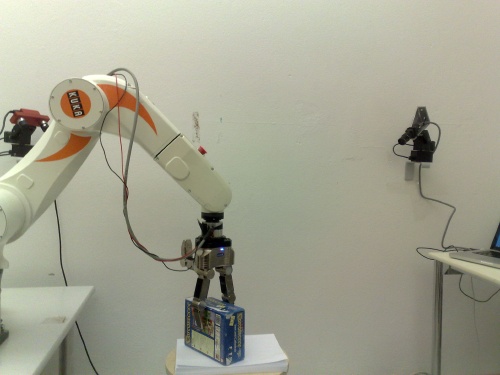
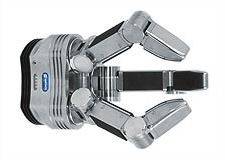
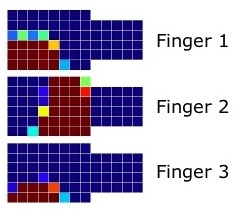
We aim to establish the likelihood of success of a grasp before attempting to lift an object. Our agent learns and memorizes what it feels like to grasp objects from various sides. Tactile data are recorded once the hand is fully closed around the object. As the object often moves while the hand is closing around it, we track the object pose throughout the grasp, and record the pose once the hand is fully closed. The robot lifts up the object and turns it upside-down. If the object stays rigidly bound to the hand during this movement, the grasp is considered successful. During training, the agent encounters both successful and unsuccessful grasps, which provide it with input-output pairs, in the form of tactile imprints and relative object-gripper configurations (input) and success/failure labels (output). These data are used to train a classifier, which is subsequently used to decide whether a grasp feels stable enough to proceed to lifting the object.
Our experiment demonstrates that joint tactile and pose-based perceptions carry valuable grasp-related information, as models trained on both hand poses and tactile parameters perform better than the models trained exclusively on one perceptual input.