NESP
Neural Estimation of Spacecraft Pose.
![]() |
Funded by Service public de Wallonie (SPW). |
![]() |
Funded by Aerospacelab. |
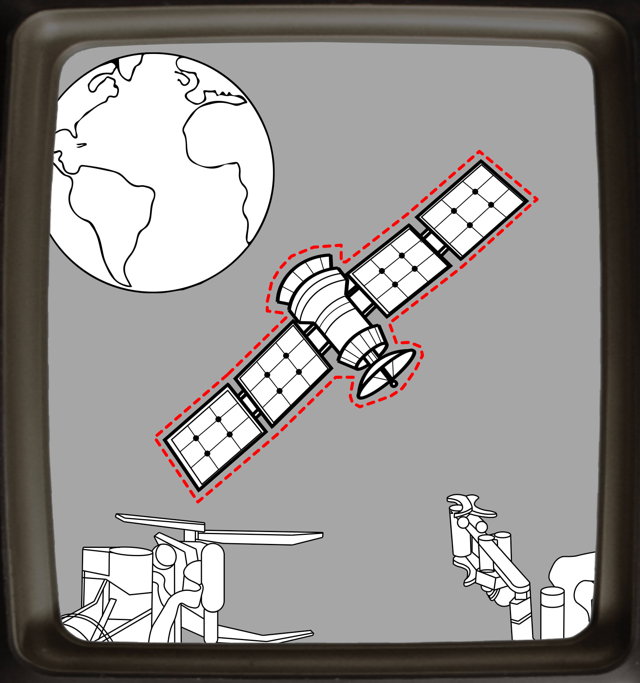
Neural Estimation of Spacecraft Pose.
![]() |
Funded by Service public de Wallonie (SPW). |
![]() |
Funded by Aerospacelab. |
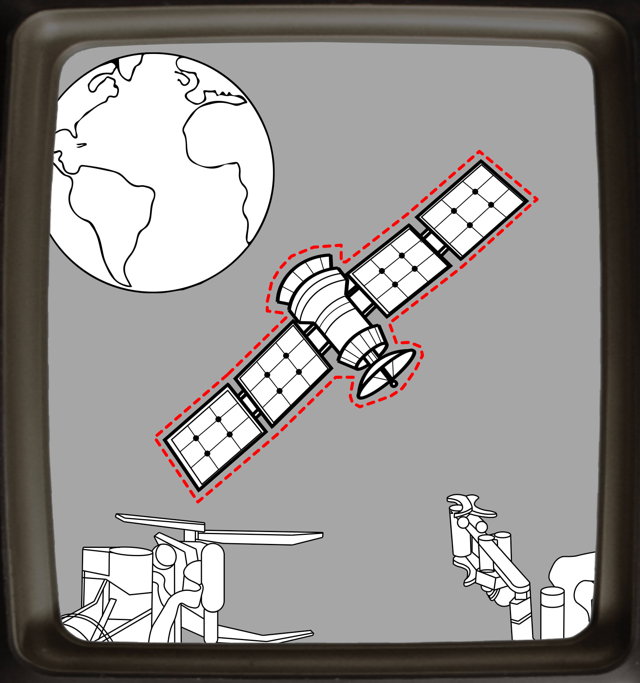
We study the problem of estimating the pose of a known spacecraft in orbital lighting conditions. Building on the state of art in terrestrial application, we specifically focus on a model that solves the problem in an end-to-end fashion, which delivers high accuracy at low computational cost, thanks to parallelizability.
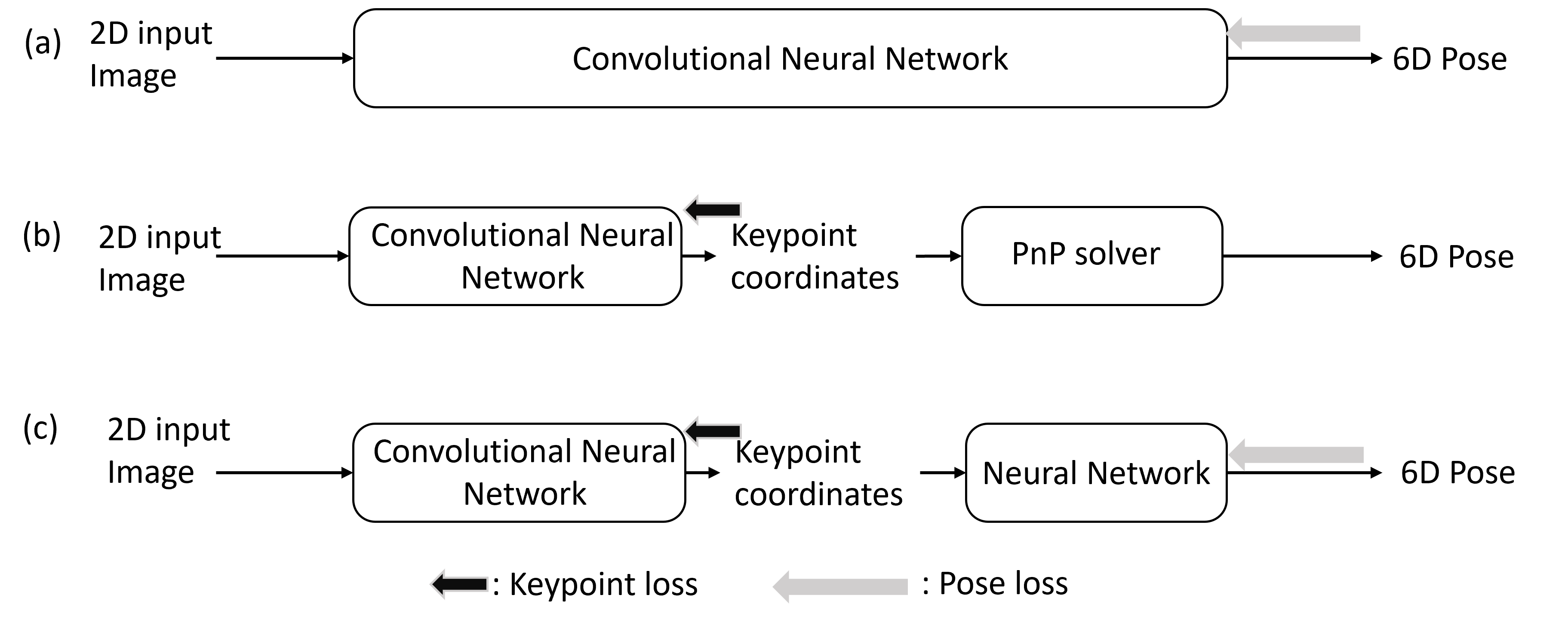
State-of-the-art methods for estimating the pose of spacecrafts in Earth-orbit images rely on a convolutional neural network either to directly regress the spacecraft’s 6D pose parameters, or to localize pre-defined keypoints that are then used to compute pose through a Perspective-n-Point solver. In our first paper (Legrand et al., 2023), we study an alternative solution that uses a convolutional network to predict keypoint locations, which are in turn used by a second network to infer the spacecraft’s 6D pose. This formulation retains the performance advantages of keypoint-based methods, while affording end-to-end training and faster processing. Our paper is the first to evaluate the applicability of such a method to the space domain. On the SPEED dataset, our approach achieves a mean rotation error of 4.69° and a mean translation error of 1.59% with a throughput of 31 fps. We show that computational complexity can be reduced at the cost of a minor loss in accuracy.