RoBétArmé
Autonomous robotic concrete projection.
![]() ![]() |
Funded by the European Union. Views and opinions expressed are however those of the author(s) only and do not necessarily reflect those of the European Union or HADEA. Neither the European Union nor the granting authority can be held responsible for them.
|
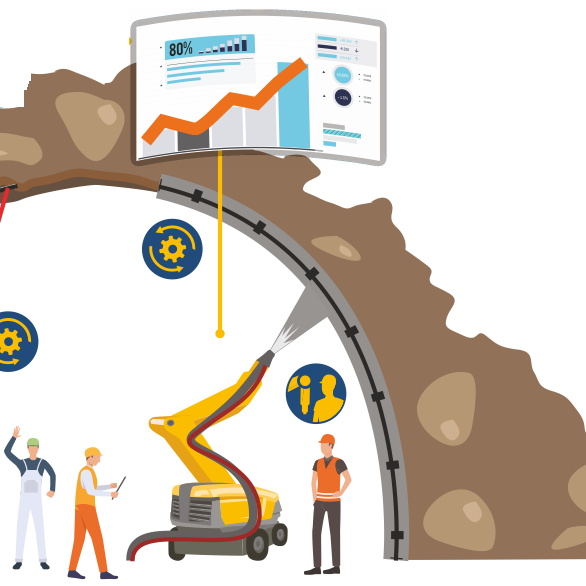
Autonomous robotic concrete projection.
![]() ![]() |
Funded by the European Union. Views and opinions expressed are however those of the author(s) only and do not necessarily reflect those of the European Union or HADEA. Neither the European Union nor the granting authority can be held responsible for them.
|
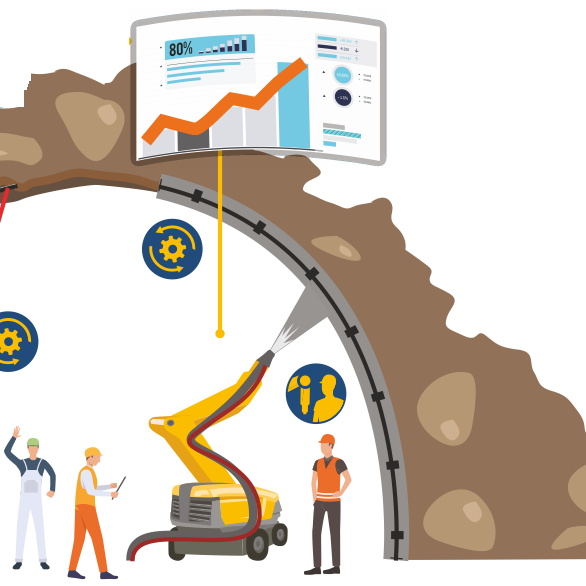
The RoBétArmé project is set to transform the construction industry by automating tough, labor-intensive tasks in shotcrete application, which involves spraying concrete onto surfaces like walls and tunnels. This process is critical for both constructing new structures and repairing old ones. KU Leuven is contributing planning and control methods for both the shotcrete application and the precision finishing of concrete surfaces, including tasks like smoothing and leveling. Backed by the European Commission’s Horizon program, this 42-month project aims to make shotcreting faster, greener, and more efficient, driving innovation in the Construction 4.0 era.
Shotcrete Planning and Control
We develop planning and control systems for shotcrete application in harsh environments, where visibility is severely impaired by dust and occlusions (Du et al., 2024). Our team is working on guiding the trajectory of the shotcrete nozzle using data-driven approaches that can handle noisy and incomplete observations from cameras. By leveraging techniques like deep reinforcement learning and uncertainty-aware algorithms, we enhance the precision of the robot’s movements, ensuring accurate concrete spraying even in difficult conditions.
A key aspect of our work is managing the uncertainty that arises from these noisy inputs. We use machine learning models, such as variational inference combined with reinforcement learning, to estimate the most likely state of the environment despite missing or unclear data. This allows us to make more informed decisions about how to apply the shotcrete efficiently, improving material conservation and reducing waste. Our goal is to create a robust, adaptive system that can handle the unpredictable nature of construction sites while maintaining high-quality outcomes for both new construction and repair projects.
The videos below illustrate some of the concepts we’re developing. Publication pending, more detail coming soon.
Robot Manipulation of Amorphous Materials
We are also developing techniques for smoothing and finishing concrete surfaces after the shotcrete process is completed. Using a robot arm, our system automates the delicate task of leveling and refining the concrete surface to meet the required smoothness. This includes developing a visual smoothness estimator, which helps the robot assess the surface condition in real-time and adjust its actions accordingly. The robot can adapt its movements based on this feedback, ensuring consistent and high-quality surface finishes, even in challenging environments where human intervention may be limited.
Our approach involves a layered control architecture (Hanut et al., 2024) that integrates perception, control, and task knowledge. By utilizing a combination of skills for surface smoothing, the robot system can execute specific actions like flattening uneven areas or fine-tuning the surface texture. This architecture allows the robot to adapt to the unpredictable nature of concrete as it settles, improving the overall efficiency and precision of the finishing process. Our contribution helps streamline the final stages of shotcrete application, enhancing both the quality and speed of construction work.
References
-
InproceedingsSkill-based Layered Control Architecture for the Robotic Manipulation of Amorphous Materials.In 43rd Benelux Meeting on Systems and Control, 2024.