Feature Hierarchies
Learning of multi-Dimensional, multi-modal features for robotic grasping.
![]() |
Funded by the Belgian National Fund for Scientific Research (FRS-FNRS). |
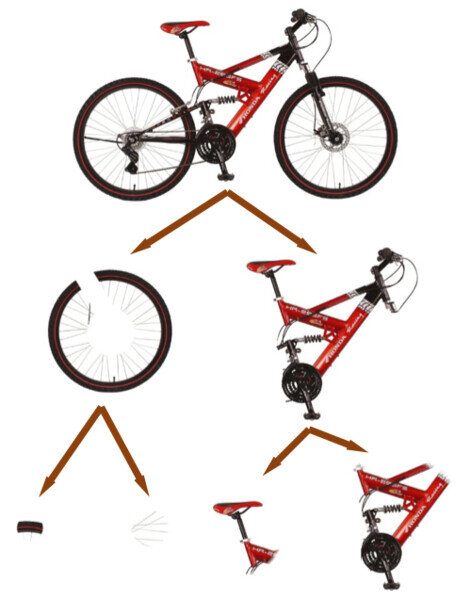
Learning of multi-Dimensional, multi-modal features for robotic grasping.
![]() |
Funded by the Belgian National Fund for Scientific Research (FRS-FNRS). |
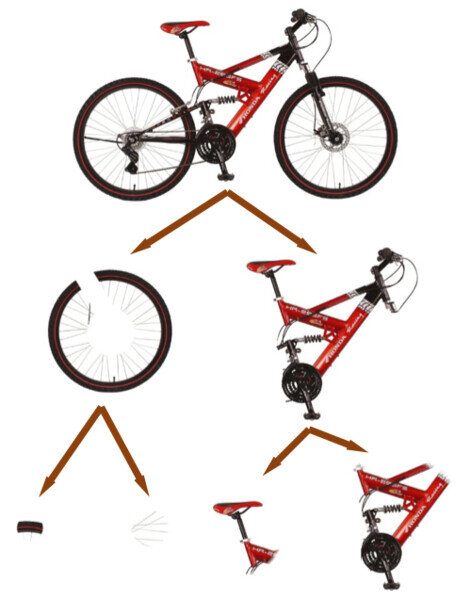
This project addresses 3D object modeling and object grasping.
Hierarchical 3D Object Models
This work introduces a generative object model for 6D pose estimation in stereo views of cluttered scenes (Detry et al., 2009). We model an object as a hierarchy of increasingly expressive object parts, where parts represent the 3D geometry and appearance of object edges. At the bottom of the hierarchy, each part encodes the spatial distribution of short segments of object edges of a specific color. Higher-level parts are formed by recursively combining more elementary parts together, the top-level part representing the whole object. The hierarchy is encoded in a Markov random field whose edges parametrize relative part configurations.
Pose inference is implemented with generic probability and machine learning techniques including belief propagation, Monte Carlo integration, and kernel density estimation. The model is learned autonomously from a set of segmented views of an object. A 3D object model is a useful asset in the context of robotic grasping, as it allows for aligning a grasp model to arbitrary object positions and orientations. Several aspects of this work are inspired by biological examples, which makes it a good building block for cognitive robotic platforms.
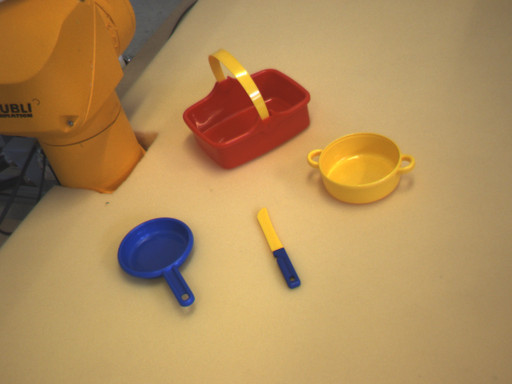
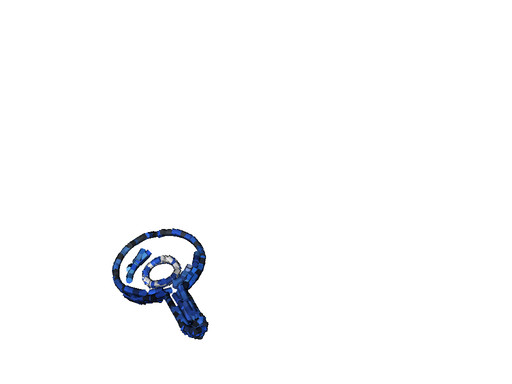
Grasp Densities
Here, we study means of modeling and learning object grasp affordances, i.e., relative object-gripper poses that lead to stable grasps. Affordances are represented probabilistically with grasp densities (Detry et al., 2011), which correspond to continuous density functions defined on the space of 6D gripper poses – 3D position and orientation.
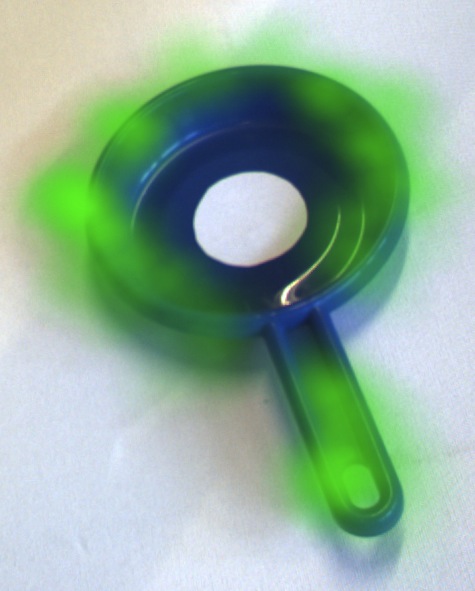
Grasp densities are linked to visual stimuli through registration with a visual model of the object they characterize, which allows the robot to grasp objects lying in arbitrary poses: to grasp an object, the object’s model is visually aligned to the correct pose; the aligned grasp density is then combined to reaching constraints to select the maximum-likelihood achievable grasp. Grasp densities are learned and refined through exploration: grasps sampled randomly from a density are performed, and an importance-sampling algorithm learns a refined density from the outcomes of these experiences. Initial grasp densities are computed from the visual model of the object.
We demonstrated that grasp densities can be learned autonomously from experience. Our experiment showed that through learning, the robot becomes increasingly efficient at inferring grasp parameters from visual evidence. The experiment also yielded conclusive results in practical scenarios where the robot needs to repeatedly grasp an object lying in an arbitrary pose, where each pose imposes a specific reaching constraint, and thus forces the robot to make use of the entire grasp density to select the most promising achievable grasp. This work led to publications in the fields of robotics (Detry et al., 2010; Detry et al., 2010; Detry et al., 2011) and developmental learning (Detry et al., 2009).